The MIDOG 2025 challenge builds on the success of previous iterations (MIDOG 2021 and MIDOG 2022) and aims to advance the field of AI-assisted cancer diagnosis. This year, the challenge focuses on two critical tasks in histopathological image analysis: mitotic figure detection and atypical mitotic figure classification. These tasks address key challenges in cancer assessment and are essential for improving the robustness and clinical applicability of AI algorithms in routine pathology workflows.
Mitotic figures, which represent cells undergoing division, are a fundamental feature in the histopathological evaluation of tumors. Their accurate detection is critical for assessing tumor proliferation activity, a key parameter in cancer grading and prognostication. Additionally, the classification of mitotic figures into normal and atypical categories provides important insights into tumor biology, as atypical mitotic figures are associated with disrupted cell division processes and are indicative of more aggressive tumor phenotypes.
Despite their importance, mitotic figure detection and atypical mitotic figure classification remain highly challenging. The variability in tissue morphology across histological samples, combined with the morphological overlap between mitotic figures and other structures, complicates accurate detection. Atypical mitotic figures further increase the complexity due to their subtle morphological differences from normal mitotic figures, which are difficult to discern even for experienced pathologists. Addressing these challenges is critical for the development of AI systems capable of supporting pathologists in delivering accurate and reproducible cancer diagnoses.
The MIDOG 2025 challenge introduces two tracks to address these challenges:
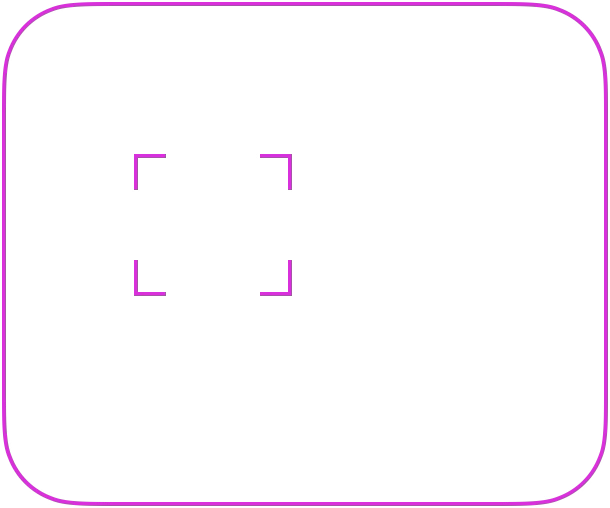
Detection
Detect Mitotic Figures in Arbitrary Tumor Tissue
In the first track of the challenge, participants are tasked with developing an algorithm for the accurate detection of mitotic figures across a wide range of tissue regions. This includes not only tumor hotspot areas but also more challenging regions such as non-tumor areas, inflamed tissue, and necrotic zones. The goal is to ensure robust performance while minimizing false positives, addressing the variability and complexity of real-world histopathological samples.
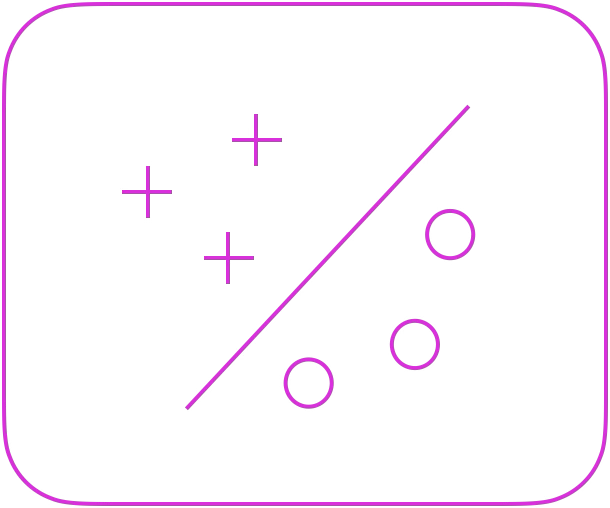
Classification
Decide if the Mitotic Figure is Atypical or Normal
In the second track, participants must develop an algorithm to classify mitotic figures into normal and atypical categories. This task aims to provide deeper insights into cancer aggressiveness, as atypical mitotic figures are associated with disrupted cell division and more aggressive tumor phenotypes. Accurate classification is critical for advancing the understanding of tumor biology and improving prognostic assessments.